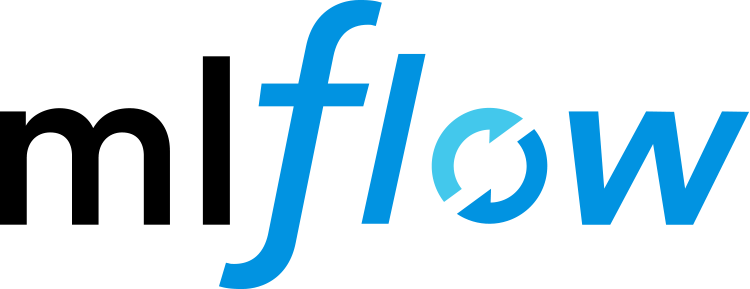
MLFlow: A platform to streamlining Machine Learning Workflows
At BlablaConf, I had the privilege to give a talk all about MLflow, a handy tool that helps make machine learning projects run smoother. I broke down how it keeps track of different experiments you do, how it bundles up your models neatly, and how it makes deploying them a breeze. I stressed how important it is for teams to work well together in data science, and MLflow makes that happen by keeping everything organized and reproducible....